In the realm of Human Resources, predictive analytics represents a revolutionary approach to gleaning insights from historical data, by utilising patterns and trends within existing information to project future outcomes, transforming HR operations into a data-driven engine of strategic foresight.
By leveraging predictive analytics in HR, organisations can make informed decisions that optimise their talent management procedures and workforce operations.
Impact on HR
The influence of predictive analytics in HR extends across multiple facets.
It serves as a critical tool for strategic HR decisions, allowing HR departments to forecast scenarios like employee turnover, recruitment success, and even performance outcomes. Such capabilities enable organisations not only to react wiser and quicker but to also anticipate challenges before they manifest.
With predictive analytics, companies can achieve a competitive advantage by enhancing retention strategies, refining the hiring process, and aligning employee initiatives with corporate goals.
Moreover, this analytics approach enables HR leaders to shift from conventional roles to strategic partners in business success. With only 17% of companies effectively utilising HR data, the potential for return on investment in analytics is tremendous.
By employing predictive analytics, organisations unlock opportunities to enhance operational efficiency and streamline decisions, all while maintaining employee satisfaction and optimising resource allocation.
Core components
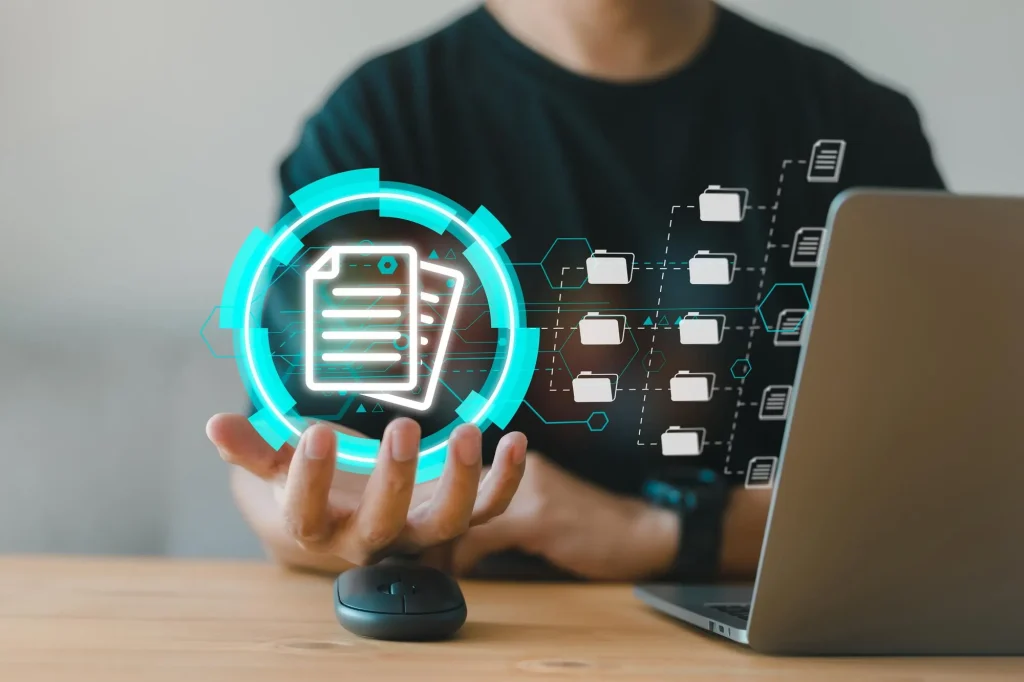
At the heart of HR predictive analytics lies a trio of essential components that drive its effectiveness in transforming workforce operations:
- Data collection: The process begins with gathering comprehensive HR data from various sources, such as employee records, performance metrics, and recruitment data. This data serves as the foundation for building predictive models.
- Model building: Utilising statistical techniques like regression analysis and machine learning, predictive models are crafted to interpret data patterns and project future outcomes. Models like decision trees help in visualising potential scenarios and their implications.
- Outcome Analysis: By evaluating the predictions against actual results, organisations can refine their models and improve accuracy over time. This feedback loop ensures continuous enhancement of the predictive capabilities, optimising the management of human resources.
Benefits of HR predictive analytics
Implementing predictive analytics in the human resources sector yields a host of advantages that elevate organisational performance and strategic decision-making abilities:
- Improved decision-making: By relying on predictive analytics, HR professionals can make informed, data-driven decisions that align closely with organisational goals, reducing reliance on intuition.
- Recruitment efficiency: Predictive models identify candidates with higher chances of long-term success, streamlining the recruitment process and ensuring the acquisition of top talent efficiently.
- Enhanced employee retention: Predictive HR analytics help identify flight risks, enabling interventions before resignations occur. This approach not only reduces turnover costs but also maintains team continuity and morale.
- Operational optimisation: With analytics insights, HR processes become more efficient, allowing professionals to focus on strategic initiatives.
- Effective turnover management: By analysing the data on employee exits, organisations can develop targeted strategies to address underlying issues and improve retention rates.
Through these benefits, predictive analytics in HR not only optimises talent management but also ensures a proactive stance in dealing with organisational challenges, paving the way for smarter HR practices.
Implementation steps
Embarking on the journey of integrating HR predictive analytics requires a structured approach. The following implementation steps will guide HR departments in successfully adopting these transformative analytics techniques:
- Define objectives: Establish clear goals for predictive analytics in HR. Determine what key areas, such as reduction in employee turnover rates or enhancements in recruitment, you want to target with analytics insights.
- Data collection and assessment: Gather relevant HR data from existing systems. This could include data on employee performance, turnover history, and recruitment processes. Ensure the data is clean, comprehensive, and accessible.
- Select appropriate tools: Choose analytics tools that not only fit the organisational needs but also align with existing infrastructure.
- Build predictive models: Engage with data scientists to develop predictive models using techniques such as decision trees and regression analysis. Such models can assist in identifying trends and predicting outcomes.
- Kiểm tra và xác nhận: Begin with a pilot project to test the models. Validate predictions against actual outcomes to refine the models continually.
- Integration and scaling: Once validated, integrate the analytics processes into everyday HR operations. Scale the models to cover broader organisational aspects.
- Continuous monitoring: Regularly assess the effectiveness of the predictive models and update them with new data. This ensures continual improvements in prediction accuracy.
Best practices for ethical data use
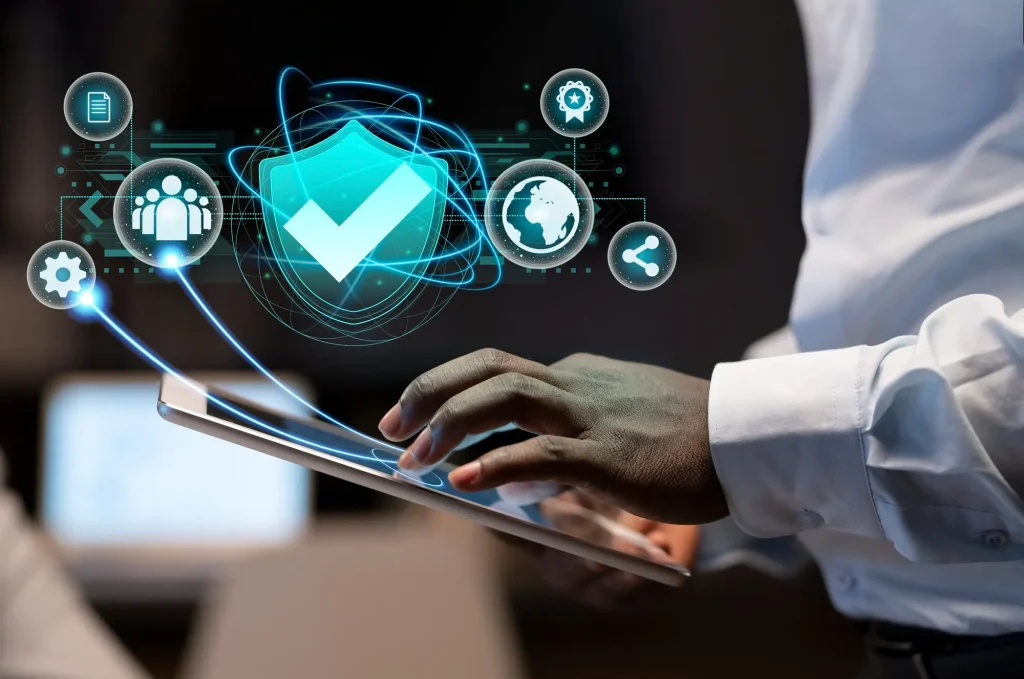
Utilising predictive HR analytics comes with responsibilities regarding ethical data use. Here are some best practices:
- Data privacy and security: Implement strong data security measures to protect employee information and comply with regulations. Ensuring privacy is a primary concern in handling predictive analytics datasets.
- Transparency: Maintain transparency with employees about how their data is utilised in predictive analytics. This openness fosters trust and cooperation.
- Bias mitigation: Be vigilant in ensuring predictive models are free from biases that could affect certain groups unfairly. Regular audits of the algorithms should be conducted.
- Ethical decision-making: Use insights from predictive analytics to make ethical decisions that align with the organisation’s values and culture.
By implementing these steps and adhering to best practices, businesses can effectively deploy HR predictive analytics to enhance their strategic HR capabilities, drive workforce optimisation, and achieve high returns on investment.
Available tools
Human Resources professionals today have a wealth of tools at their disposal designed to facilitate effective predictive analytics in HR. Leveraging these tools can radically change how talent management and workforce optimisation are approached.
Serving as a comprehensive HR and payroll management platform, MiHCM integrates sophisticated predictive analytics capabilities to optimise talent management. Features such as HR analytics and SmartAssist provide detailed insights into performance and efficiency.
By utilising these tools, organisations can create a thorough analytics strategy that supports a range of HR decisions from employee turnover prediction to recruitment success analysis, thereby enhancing strategic HR capabilities.
Techniques in HR predictive analytics
HR predictive analytics are not only about the toolset, but also the techniques deployed to get meaningful insights. Several analytical methods are employed by HR professionals, including:
- Regression analysis: This technique allows HR teams to forecast outcomes by examining relationships between variables, such as employee satisfaction and turnover rates.
- Machine learning models: By applying machine learning algorithms, organisations can develop models that predict future behaviours such as performance trends and attrition. These models offer automatic learning capabilities and are crucial for high-volume HR data analysis.
- Decision trees: These are particularly helpful for visualising possible outcomes and honing HR strategies. They allow HR practitioners to test different scenarios and make data-driven decisions.
- Cluster analysis: This method groups similar data points, aiding in identifying patterns such as common reasons for employee disengagement or attrition risks.
The proper application of these techniques results in improved HR outcomes, such as better recruitment processes, reduced turnover, and optimised workforce planning.
By marrying these analytical methods with comprehensive tools, HR departments can effectively transition into a data-centric role, significantly enhancing their capacity for strategic decision making.
Case Study 1: HP
HP’s utilisation of HR predictive analytics is a stellar example of how data-driven strategies can substantially cut costs and improve workforce management.
By developing a predictive model that generated a Flight Risk score for their employees, HP successfully managed to identify those who were likely to leave the company.
The proactive steps taken based on these insights led to a dramatic reduction in their employee turnover rate, saving the company approximately $300 million. HP’s approach underscores the potential of predictive analytics for effectively managing and predicting employee turnover.
Case Study 2: Google
Google is a trailblazer in leveraging predictive models to fine-tune its recruitment and retention processes.
By marrying algorithms capable of predicting employee retention with automated recruitment systems, Google has streamlined its hiring procedures. The outcome is a significant improvement in aligning the recruitment strategies with desired job outcomes and a robust employee retention rate.
This case illustrates how predictive HR analytics can optimise talent acquisition efforts, bringing efficiency to the forefront of HR practices.
Case Study 3: Best Buy
Best Buy offers an insightful look into how predictive analytics can transform operational strategies.
It discovered that a mere 0.1% increase in employee engagement could boost store revenue by $100,000 annually. This revelation prompted the company to establish proactive measures aimed at elevating employee engagement, thereby directly enhancing both morale and revenue.
Such tangible results attest to the power of data-driven decision-making, emphasising how predictive analytics in HR plays a vital role in linking workforce dynamics to organisational performance.
These case studies demonstrate the profound impact of predictive analytics on workforce optimisation and talent management. From effectively managing turnover with tools like turnover management to improving recruitment processes and raising employee engagement, predictive analytics enables smarter strategic decisions across various HR functions.
The benefits, ranging from enhanced recruitment efficiency to streamlined turnover management, highlight the transformative potential of predictive analytics, making a compelling case for its broader adoption across industries.
With solutions such as the MiHCM suite, businesses are empowered to fortify their HR predictive analytics capabilities by automating routine HR tasks, allowing more focus on analytical insights that drive better workforce outcomes.
Enhancing retention
Incorporating predictive analytics within HR processes holds transformative potential, especially in enhancing employee retention rates.
By analysing historical data, organisations can predict which employees are at risk of leaving. These predictions allow HR professionals to implement targeted retention strategies to prevent costly turnover.
By honing in on the warning signs – such as declining engagement or changes in productivity – HR can act proactively to address them, moving from reactive to proactive management.
Predicting performance
Performance prediction is another cornerstone of predictive HR analytics.
By utilising techniques like regression analysis and machine learning models, organisations can foresee how employees might perform in future scenarios based on their past and present data.
Predicting employee performance allows HR teams to allocate training resources where they’re needed most and forecast potential leadership trajectories.
For example, MiHCM equips HR teams with tools to dissect performance metrics comprehensively. This approach encourages a seamless transition of underperformers to high achievers by moulding performance enhancement strategies that are as precise as they are effective.
Through predictive workforce analytics, organisations achieve a substantial head start in strategically managing both retention and performance. This strategic foresight nurtures a workplace culture where employees are more engaged, aligned with the organisational mission, and less likely to leave, creating a beneficial cycle of retention and performance improvement.
Emerging trends
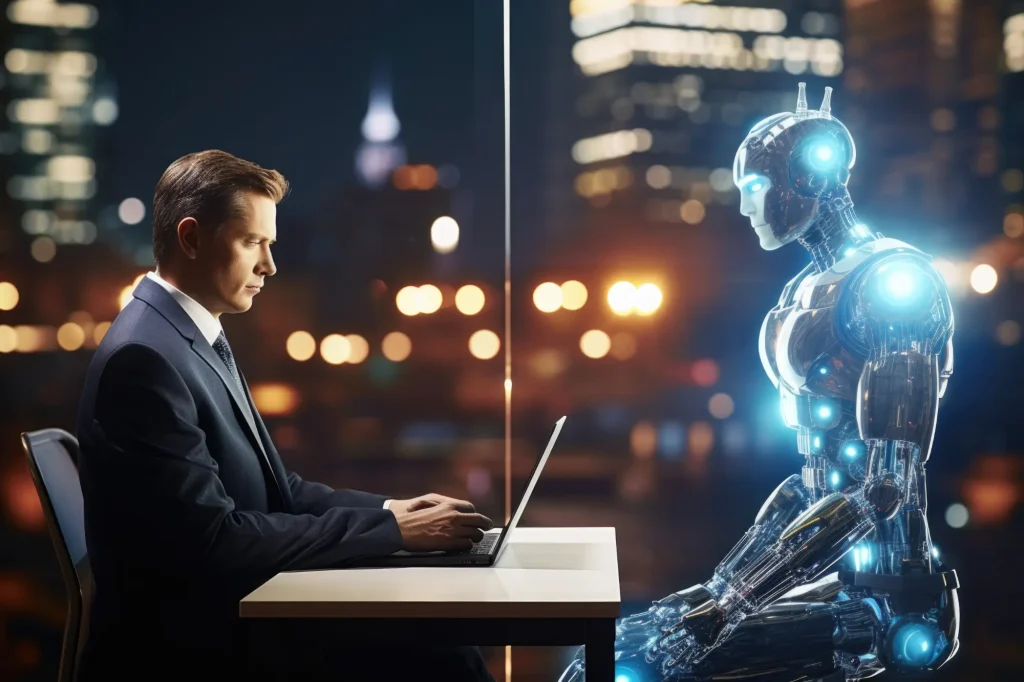
The field of HR predictive analytics is rapidly advancing, driven by technological innovation and the increasing need for strategic workforce management.
A notable trend is the integration of Artificial Intelligence (AI) with predictive analytics, which enables more accurate data processing and predictive modelling. AI-powered analytics platforms can now analyse vast datasets with precision, uncovering insights that were previously unattainable.
Another trend is the shift towards real-time analytics, allowing HR teams to make informed decisions on-the-fly.
Real-time data processing helps organisations to swiftly adapt their strategies to changing employee dynamics and market conditions. Additionally, there is an increasing emphasis on personalising employee experiences.
By leveraging predictive analytics, companies can tailor their HR practices to meet individual employee needs, enhancing engagement and satisfaction.
Addressing challenges
Despite the promising trends, implementing predictive HR analytics poses significant challenges.
Data privacy and ethical use remain at the forefront. Organisations must navigate complex data protection regulations while ensuring that their analytics practices are transparent and equitable. This necessitates comprehensive data governance frameworks to safeguard employee information and maintain trust.
Another challenge is the technical expertise required to leverage predictive analytics effectively.
Many HR departments may lack the necessary skills in data science and analytics, which can hinder the adoption and integration of sophisticated tools and techniques. Investing in training and development is crucial for building internal competencies and ensuring successful analytics implementation.
Finally, there is the hurdle of change management.
Transitioning to a data-driven HR approach often requires a cultural shift within organisations. Stakeholders may need to be educated on the value of predictive analytics and how it contributes to achieving strategic goals. Building a data-driven culture where decisions are consistently informed by analytics insights can foster more effective and agile HR practices.
While predictive analytics in HR offers immense potential for transforming workforce management and optimising talent strategies, organisations must address these challenges to fully realise its benefits.
By staying abreast of emerging trends and developing robust strategies to overcome obstacles, HR leaders can harness the power of predictive analytics to stay competitive in an increasingly complex business environment.